To create a blocking search and display properties of the job. Running a blocking search creates a search job and runs the search synchronously in 'blocking' mode. The job is returned after the search has finished and all the results are in. When you create a search job, you need to set the parameters of the job as a dictionary of key-value pairs. Setting the slice to a python date object: job.setall(time(10, 2)) job.setall(date(2000, 4, 2)) job.setall(datetime(2000, 4, 2, 10, 2)) Run a jobs command. Running the job here will not effect it’s existing schedule with another crontab process: jobstandardoutput = job.run Creating a job with a comment.
- Python Job Runners
- Python Job Runner
- Python Job Runner Online
- Python Job Runner Download
- Python Job Runner Tutorial
The Apache Flink Runner can be used to execute Beam pipelines using ApacheFlink. For execution you can choose between a clusterexecution mode (e.g. Yarn/Kubernetes/Mesos) or a local embedded execution modewhich is useful for testing pipelines.
The Flink Runner and Flink are suitable for large scale, continuous jobs, and provide:
- A streaming-first runtime that supports both batch processing and data streaming programs
- A runtime that supports very high throughput and low event latency at the same time
- Fault-tolerance with exactly-once processing guarantees
- Natural back-pressure in streaming programs
- Custom memory management for efficient and robust switching between in-memory and out-of-core data processing algorithms
- Integration with YARN and other components of the Apache Hadoop ecosystem
It is important to understand that the Flink Runner comes in two flavors:
Python Job Runners
- The original classic Runner which supports only Java (and other JVM-based languages)
- The newer portable Runner which supports Java/Python/Go
You may ask why there are two Runners?
Beam and its Runners originally only supported JVM-based languages(e.g. Java/Scala/Kotlin). Python and Go SDKs were added later on. Thearchitecture of the Runners had to be changed significantly to support executingpipelines written in other languages.
If your applications only use Java, then you should currently go with the classicRunner. Eventually, the portable Runner will replace the classic Runner becauseit contains the generalized framework for executing Java, Python, Go, and morelanguages in the future.
If you want to run Python pipelines with Beam on Flink you want to use theportable Runner. For more information onportability, please visit the Portability page.
Consequently, this guide is split into parts to document the classic andthe portable functionality of the Flink Runner.In addition, Python provides convenience wrappers to handle the full lifecycle of the runner,and so is further split depending on whether to manage the portabilitycomponents automatically (recommended) or manually.Please use the switcher below to select the appropriate mode for the Runner:
Prerequisites and Setup
If you want to use the local execution mode with the Flink Runner you don’t haveto complete any cluster setup. You can simply run your Beam pipeline. Be sure toset the Runner to FlinkRunner
PortableRunner
.
To use the Flink Runner for executing on a cluster, you have to setup a Flink cluster by following theFlink Setup Quickstart.
Dependencies
You must specify your dependency on the Flink Runnerin your pom.xml
or build.gradle
. Use the Beam version and the artifact idfrom the above table. For example:
You will need Docker to be installed in your execution environment.To run an embedded flink cluster or use the Flink runner for Python < 3.6you will also need to have java available in your execution environment.
You will need Docker to be installed in your execution environment.
Executing a Beam pipeline on a Flink Cluster
For executing a pipeline on a Flink cluster you need to package your programalong with all dependencies in a so-called fat jar. How you do this depends onyour build system but if you follow along the Beam Quickstart this is the command that you have to run:
Look for the output JAR of this command in thetarget
folder.
The Beam Quickstart Maven project is setup to use the Maven Shade plugin tocreate a fat jar and the -Pflink-runner
argument makes sure to include thedependency on the Flink Runner.
For running the pipeline the easiest option is to use the flink
command whichis part of Flink:
$ bin/flink run -c org.apache.beam.examples.WordCount /path/to/your.jar–runner=FlinkRunner –other-parameters
Alternatively you can also use Maven’s exec command. For example, to execute theWordCount example:
If you have a Flink JobManager
running on your local machine you can provide localhost:8081
forflinkMaster
. Otherwise an embedded Flink cluster will be started for the job.
To run a pipeline on Flink, set the runner to FlinkRunner
and flink_master
to the master URL of a Flink cluster.In addition, optionally set environment_type
set to LOOPBACK
. For example,after starting up a local flink cluster,one could run:
To run on an embedded Flink cluster, simply omit the flink_master
optionand an embedded Flink cluster will be automatically started and shut down for the job.
The optional flink_version
option may be required as well for older versions of Python.
Starting with Beam 2.18.0, pre-built Flink Job Service Docker images are available at Docker Hub:Flink 1.10,Flink 1.11,Flink 1.12.Flink 1.13.
To run a pipeline on an embedded Flink cluster:
(1) Start the JobService endpoint: docker run --net=host apache/beam_flink1.10_job_server:latest
The JobService is the central instance where you submit your Beam pipeline to.The JobService will create a Flink job for the pipeline and execute the job.
(2) Submit the Python pipeline to the above endpoint by using the PortableRunner
, job_endpoint
set to localhost:8099
(this is the default address of the JobService).Optionally set environment_type
set to LOOPBACK
. For example:
To run on a separate Flink cluster:
(1) Start a Flink cluster which exposes the Rest interface (e.g. localhost:8081
by default).
(2) Start JobService with Flink Rest endpoint: docker run --net=host apache/beam_flink1.10_job_server:latest --flink-master=localhost:8081
.

(3) Submit the pipeline as above.
Note that environment_type=LOOPBACK
is only intended for local testing,and will not work on remote clusters.See here for details.
Additional information and caveats
Monitoring your job
You can monitor a running Flink job using the Flink JobManager Dashboard or its Rest interfaces. By default, this is available at port 8081
of the JobManager node. If you have a Flink installation on your local machine that would be http://localhost:8081
. Note: When you use the [local]
mode an embedded Flink cluster will be started which does not make a dashboard available.
Streaming Execution
If your pipeline uses an unbounded data source or sink, the Flink Runner will automatically switch to streaming mode. You can enforce streaming mode by using the --streaming
flag.
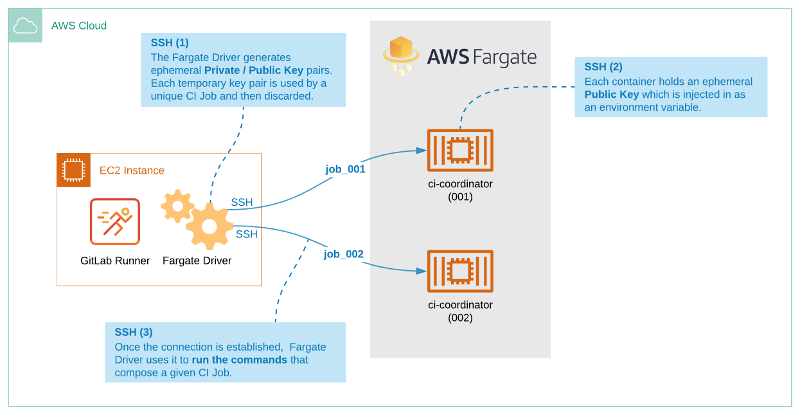
Note: The Runner will print a warning message when unbounded sources are used and checkpointing is not enabled.Many sources like PubSubIO
rely on their checkpoints to be acknowledged which can only be done when checkpointing is enabled for the FlinkRunner
. To enable checkpointing, please set checkpointingInterval
checkpointing_interval
to the desired checkpointing interval in milliseconds.
Pipeline options for the Flink Runner
When executing your pipeline with the Flink Runner, you can set these pipeline options.
The following list of Flink-specific pipeline options is generated automatically from theFlinkPipelineOptionsreference class:
allowNonRestoredState | Flag indicating whether non restored state is allowed if the savepoint contains state for an operator that is no longer part of the pipeline. | Default: false |
autoBalanceWriteFilesShardingEnabled | Flag indicating whether auto-balance sharding for WriteFiles transform should be enabled. This might prove useful in streaming use-case, where pipeline needs to write quite many events into files, typically divided into N shards. Default behavior on Flink would be, that some workers will receive more shards to take care of than others. This cause workers to go out of balance in terms of processing backlog and memory usage. Enabling this feature will make shards to be spread evenly among available workers in improve throughput and memory usage stability. | Default: false |
autoWatermarkInterval | The interval in milliseconds for automatic watermark emission. | |
checkpointTimeoutMillis | The maximum time in milliseconds that a checkpoint may take before being discarded. | Default: -1 |
checkpointingInterval | The interval in milliseconds at which to trigger checkpoints of the running pipeline. Default: No checkpointing. | Default: -1 |
checkpointingMode | The checkpointing mode that defines consistency guarantee. | Default: EXACTLY_ONCE |
disableMetrics | Disable Beam metrics in Flink Runner | Default: false |
executionModeForBatch | Flink mode for data exchange of batch pipelines. Reference {@link org.apache.flink.api.common.ExecutionMode}. Set this to BATCH_FORCED if pipelines get blocked, see https://issues.apache.org/jira/browse/FLINK-10672 | Default: PIPELINED |
executionRetryDelay | Sets the delay in milliseconds between executions. A value of {@code -1} indicates that the default value should be used. | Default: -1 |
externalizedCheckpointsEnabled | Enables or disables externalized checkpoints. Works in conjunction with CheckpointingInterval | Default: false |
failOnCheckpointingErrors | Sets the expected behaviour for tasks in case that they encounter an error in their checkpointing procedure. If this is set to true, the task will fail on checkpointing error. If this is set to false, the task will only decline a the checkpoint and continue running. | Default: true |
fasterCopy | Remove unneeded deep copy between operators. See https://issues.apache.org/jira/browse/BEAM-11146 | Default: false |
filesToStage | Jar-Files to send to all workers and put on the classpath. The default value is all files from the classpath. | |
finishBundleBeforeCheckpointing | If set, finishes the current bundle and flushes all output before checkpointing the state of the operators. By default, starts checkpointing immediately and buffers any remaining bundle output as part of the checkpoint. The setting may affect the checkpoint alignment. | Default: false |
flinkMaster | Address of the Flink Master where the Pipeline should be executed. Can either be of the form 'host:port' or one of the special values [local], [collection] or [auto]. | Default: [auto] |
latencyTrackingInterval | Interval in milliseconds for sending latency tracking marks from the sources to the sinks. Interval value <= 0 disables the feature. | Default: 0 |
maxBundleSize | The maximum number of elements in a bundle. | Default: 1000 |
maxBundleTimeMills | The maximum time to wait before finalising a bundle (in milliseconds). | Default: 1000 |
maxParallelism | The pipeline wide maximum degree of parallelism to be used. The maximum parallelism specifies the upper limit for dynamic scaling and the number of key groups used for partitioned state. | Default: -1 |
minPauseBetweenCheckpoints | The minimal pause in milliseconds before the next checkpoint is triggered. | Default: -1 |
numConcurrentCheckpoints | The maximum number of concurrent checkpoints. Defaults to 1 (=no concurrent checkpoints). | Default: 1 |
numberOfExecutionRetries | Sets the number of times that failed tasks are re-executed. A value of zero effectively disables fault tolerance. A value of -1 indicates that the system default value (as defined in the configuration) should be used. | Default: -1 |
objectReuse | Sets the behavior of reusing objects. | Default: false |
parallelism | The degree of parallelism to be used when distributing operations onto workers. If the parallelism is not set, the configured Flink default is used, or 1 if none can be found. | Default: -1 |
reIterableGroupByKeyResult | Flag indicating whether result of GBK needs to be re-iterable. Re-iterable result implies that all values for a single key must fit in memory as we currently do not support spilling to disk. | Default: false |
reportCheckpointDuration | If not null, reports the checkpoint duration of each ParDo stage in the provided metric namespace. | |
retainExternalizedCheckpointsOnCancellation | Sets the behavior of externalized checkpoints on cancellation. | Default: false |
savepointPath | Savepoint restore path. If specified, restores the streaming pipeline from the provided path. | |
shutdownSourcesAfterIdleMs | Shuts down sources which have been idle for the configured time of milliseconds. Once a source has been shut down, checkpointing is not possible anymore. Shutting down the sources eventually leads to pipeline shutdown (=Flink job finishes) once all input has been processed. Unless explicitly set, this will default to Long.MAX_VALUE when checkpointing is enabled and to 0 when checkpointing is disabled. See https://issues.apache.org/jira/browse/FLINK-2491 for progress on this issue. | Default: -1 |
stateBackend | State backend to store Beam's state. Use 'rocksdb' or 'filesystem'. | |
stateBackendFactory | Sets the state backend factory to use in streaming mode. Defaults to the flink cluster's state.backend configuration. | |
stateBackendStoragePath | State backend path to persist state backend data. Used to initialize state backend. |
allow_non_restored_state | Flag indicating whether non restored state is allowed if the savepoint contains state for an operator that is no longer part of the pipeline. | Default: false |
auto_balance_write_files_sharding_enabled | Flag indicating whether auto-balance sharding for WriteFiles transform should be enabled. This might prove useful in streaming use-case, where pipeline needs to write quite many events into files, typically divided into N shards. Default behavior on Flink would be, that some workers will receive more shards to take care of than others. This cause workers to go out of balance in terms of processing backlog and memory usage. Enabling this feature will make shards to be spread evenly among available workers in improve throughput and memory usage stability. | Default: false |
auto_watermark_interval | The interval in milliseconds for automatic watermark emission. | |
checkpoint_timeout_millis | The maximum time in milliseconds that a checkpoint may take before being discarded. | Default: -1 |
checkpointing_interval | The interval in milliseconds at which to trigger checkpoints of the running pipeline. Default: No checkpointing. | Default: -1 |
checkpointing_mode | The checkpointing mode that defines consistency guarantee. | Default: EXACTLY_ONCE |
disable_metrics | Disable Beam metrics in Flink Runner | Default: false |
execution_mode_for_batch | Flink mode for data exchange of batch pipelines. Reference {@link org.apache.flink.api.common.ExecutionMode}. Set this to BATCH_FORCED if pipelines get blocked, see https://issues.apache.org/jira/browse/FLINK-10672 | Default: PIPELINED |
execution_retry_delay | Sets the delay in milliseconds between executions. A value of {@code -1} indicates that the default value should be used. | Default: -1 |
externalized_checkpoints_enabled | Enables or disables externalized checkpoints. Works in conjunction with CheckpointingInterval | Default: false |
fail_on_checkpointing_errors | Sets the expected behaviour for tasks in case that they encounter an error in their checkpointing procedure. If this is set to true, the task will fail on checkpointing error. If this is set to false, the task will only decline a the checkpoint and continue running. | Default: true |
faster_copy | Remove unneeded deep copy between operators. See https://issues.apache.org/jira/browse/BEAM-11146 | Default: false |
files_to_stage | Jar-Files to send to all workers and put on the classpath. The default value is all files from the classpath. | |
finish_bundle_before_checkpointing | If set, finishes the current bundle and flushes all output before checkpointing the state of the operators. By default, starts checkpointing immediately and buffers any remaining bundle output as part of the checkpoint. The setting may affect the checkpoint alignment. | Default: false |
flink_master | Address of the Flink Master where the Pipeline should be executed. Can either be of the form 'host:port' or one of the special values [local], [collection] or [auto]. | Default: [auto] |
latency_tracking_interval | Interval in milliseconds for sending latency tracking marks from the sources to the sinks. Interval value <= 0 disables the feature. | Default: 0 |
max_bundle_size | The maximum number of elements in a bundle. | Default: 1000 |
max_bundle_time_mills | The maximum time to wait before finalising a bundle (in milliseconds). | Default: 1000 |
max_parallelism | The pipeline wide maximum degree of parallelism to be used. The maximum parallelism specifies the upper limit for dynamic scaling and the number of key groups used for partitioned state. | Default: -1 |
min_pause_between_checkpoints | The minimal pause in milliseconds before the next checkpoint is triggered. | Default: -1 |
num_concurrent_checkpoints | The maximum number of concurrent checkpoints. Defaults to 1 (=no concurrent checkpoints). | Default: 1 |
number_of_execution_retries | Sets the number of times that failed tasks are re-executed. A value of zero effectively disables fault tolerance. A value of -1 indicates that the system default value (as defined in the configuration) should be used. | Default: -1 |
object_reuse | Sets the behavior of reusing objects. | Default: false |
parallelism | The degree of parallelism to be used when distributing operations onto workers. If the parallelism is not set, the configured Flink default is used, or 1 if none can be found. | Default: -1 |
re_iterable_group_by_key_result | Flag indicating whether result of GBK needs to be re-iterable. Re-iterable result implies that all values for a single key must fit in memory as we currently do not support spilling to disk. | Default: false |
report_checkpoint_duration | If not null, reports the checkpoint duration of each ParDo stage in the provided metric namespace. | |
retain_externalized_checkpoints_on_cancellation | Sets the behavior of externalized checkpoints on cancellation. | Default: false |
savepoint_path | Savepoint restore path. If specified, restores the streaming pipeline from the provided path. | |
shutdown_sources_after_idle_ms | Shuts down sources which have been idle for the configured time of milliseconds. Once a source has been shut down, checkpointing is not possible anymore. Shutting down the sources eventually leads to pipeline shutdown (=Flink job finishes) once all input has been processed. Unless explicitly set, this will default to Long.MAX_VALUE when checkpointing is enabled and to 0 when checkpointing is disabled. See https://issues.apache.org/jira/browse/FLINK-2491 for progress on this issue. | Default: -1 |
state_backend | State backend to store Beam's state. Use 'rocksdb' or 'filesystem'. | |
state_backend_factory | Sets the state backend factory to use in streaming mode. Defaults to the flink cluster's state.backend configuration. | |
state_backend_storage_path | State backend path to persist state backend data. Used to initialize state backend. |
For general Beam pipeline options see thePipelineOptionsreference.
Flink Version Compatibility
The Flink cluster version has to match the minor version used by the FlinkRunner.The minor version is the first two numbers in the version string, e.g. in 1.13.0
theminor version is 1.13
.
We try to track the latest version of Apache Flink at the time of the Beam release.A Flink version is supported by Beam for the time it is supported by the Flink community.The Flink community supports the last two minor versions. When support for a Flink version is dropped, it may be deprecated and removed also from Beam.To find out which version of Flink is compatible with Beam please see the table below:
Beam Version | Flink Version | Artifact Id |
---|---|---|
≥ 2.31.0 | 1.13.x * | beam-runners-flink-1.13 |
1.12.x * | beam-runners-flink-1.12 | |
1.11.x * | beam-runners-flink-1.11 | |
2.30.0 | 1.12.x * | beam-runners-flink-1.12 |
1.11.x * | beam-runners-flink-1.11 | |
1.10.x | beam-runners-flink-1.10 | |
2.27.0 - 2.29.0 | 1.12.x * | beam-runners-flink-1.12 |
1.11.x * | beam-runners-flink-1.11 | |
1.10.x | beam-runners-flink-1.10 | |
1.9.x | beam-runners-flink-1.9 | |
1.8.x | beam-runners-flink-1.8 | |
2.25.0 - 2.26.0 | 1.11.x * | beam-runners-flink-1.11 |
1.10.x | beam-runners-flink-1.10 | |
1.9.x | beam-runners-flink-1.9 | |
1.8.x | beam-runners-flink-1.8 | |
2.21.0 - 2.24.0 | 1.10.x | beam-runners-flink-1.10 |
1.9.x | beam-runners-flink-1.9 | |
1.8.x | beam-runners-flink-1.8 | |
2.17.0 - 2.20.0 | 1.9.x | beam-runners-flink-1.9 |
1.8.x | beam-runners-flink-1.8 | |
1.7.x | beam-runners-flink-1.7 | |
2.13.0 - 2.16.0 | 1.8.x | beam-runners-flink-1.8 |
1.7.x | beam-runners-flink-1.7 | |
1.6.x | beam-runners-flink-1.6 | |
1.5.x | beam-runners-flink_2.11 | |
2.10.0 - 2.16.0 | 1.7.x | beam-runners-flink-1.7 |
1.6.x | beam-runners-flink-1.6 | |
1.5.x | beam-runners-flink_2.11 | |
2.9.0 | 1.5.x | beam-runners-flink_2.11 |
2.8.0 | ||
2.7.0 | ||
2.6.0 | ||
2.5.0 | 1.4.x with Scala 2.11 | beam-runners-flink_2.11 |
2.4.0 | ||
2.3.0 | ||
2.2.0 | 1.3.x with Scala 2.10 | beam-runners-flink_2.10 |
2.1.x | ||
2.0.0 | 1.2.x with Scala 2.10 | beam-runners-flink_2.10 |
Python Job Runner
* This version does not have a published docker image for the Flink Job Service.
For retrieving the right Flink version, see the Flink downloads page.
For more information, the Flink Documentation can be helpful.
Beam Capability
The Beam Capability Matrix documents thecapabilities of the classic Flink Runner.
The Portable CapabilityMatrix documentsthe capabilities of the portable Flink Runner.
Last updated on 2021/07/09
Have you found everything you were looking for?
Was it all useful and clear? Is there anything that you would like to change? Let us know!
In this tutorial we will write a job submission script for SLURM. If you haven’t yet,you should:
- be comfortable accessing the Sherlock cluster
- understand SLURM job submission
and then move forward with this tutorial!
Scenario
Python Job Runner Online
You just finished up a really cool analysis, and you need to scale it. An HPCcluster with a job manager such as SLURM is a great way to do this! In this tutorial,we will walk through a very simple method to do this. First, let’s talk about ourstrategy for today.
- Write an executable script in R / Python
- Organize your inputs, output location, and scripts.
- Loop over some set of variables and submit a SLURM job to use your executable to process each one.
We will cover each of these steps in detail.
Write an Executable Script
You first have some script in R or Python. It likely reads in data, processes it, and creates a result. You will need to turn this script into an executable, meaning that it accepts variable arguments.
Using R
R actually makes this very easy. While there are advanced input parsers, you can retrieve your script inputs with just a few lines:
if I saved this in a script called “run.R” I could then execute:
and input1
would be assigned to “tomato,” and “potato” and “shiabato” to input2
and input3
, respectively. By the way, if you aren’t familiar with Rscript, it’s literallythe R script executable. We are going to be using it in our work today!
Python Job Runner Download
Using Python
Python is just as easy! Instead of commandArgs, we use the sys
module. Thesame would look like this:
Calling would then look like:
sys.argv
is actually just a list of your calling script and the input argumentsfollowing it. If you are keen, you’ll realize that Python starts indexing at 0, and we are skipping over the value at sys.argv[0]
. This would actually coincide to the name of your script. If you are interested in advanced input parsing, then youshould look at argparse. You can read about our example using argparse for a module entrypoint here, or go directly to the gist.
A Little About Executables
When you write your executable, it’s good practice to not hard code any variablesFor example, if my script is going to process an input file, I could take in justa subject identifier and then define the full path in the script:
But guess what? If you change a location, your script breaks. Instead, assign this path duty to the calling script (we haven’t written this yet). This means that your executable should instead expect a generalinput_path
:
Notice that for both, as a sanity check we check that input_path
exists.
Path errors are extremely common in scientific programming, and you should always do this check.
Organize inputs, outputs, scripts
You will hugely benefit if you keep your scripts and inputs / outputs organized. This generally means the following:
Scripts go in $HOME
your $HOME folder is backed up. This also means you have a stricter quota, and should use it for scripts and valuables (and not data). Under $HOME, it’s recommended to adopt a structure based on version controlled code. For example, I might have a folder $HOME/projects and inside that folder I would clone Github repositories that are relevant to my work. Everything would be commit, and if you are a pro, you would have testing. Generally,
You should be able to completely lose your entire $HOME and be OK because the code is under version control.
Data goes in $SCRATCH
$SCRATCH is a good place for temporary data outputs. If you have a more long term data storage resource (e.g., $OAK at Stanford) then you might store data here too). This means that you might have an equivalent folder setup here for project data,$SCRATCH/projects and subfolders of projects under that. If it’s a shared project between your group, you could put it in $PI_SCRATCH. For example, for my project “LizardLips” with subject folders “LizardA” and “LizardB” I might decide on this outputstructure:
Inputs
Now, arguably if you have a small input file (e.g., a csv) it would be OK to store it in your $HOME. But with all this big data I’m betting that your input data is large too. The trick here is that you want to create an organizational setup where you canalways link an input object (subject, sample, timepoint, etc.) to its output based on a unique identifier. In the data organization above, we see that our data is organized based on subjects (LizardA and LizardB) and you can imagine now having a programmatically defined input and output location for each:
With the above structure, I can have confidence that my inputs for LizardA
, if they exist, are in /scratch/users/vsochat/LizardLips/LizardA/inputs
. What do you name these folders? It’s largely up to you, and you should choose names appropriate for your data.There are many known data organization standards (e.g., BIDS for neuroimaging) and you should havea discussion with your lab mates, and (highly recommended) reach out to research computing to have a meetingto discuss a data storage strategy.
Loop submission using your executable
You then want to loop over some set of input variables (for example, csv files with data.) You can imagine doing this on your computer - each of the inputs would be processed in serial. Your time to completion, where T is the time for one script to run, and N is the number of inputs, is N * T. That can take many hours if you have a few hundredinputs each taking 10 minutes, and it’s totally unfeasible if you have thousands of simulations, each of which might need 30 minutes to an hour.
Strategy 1: Submit a Job File
As a graduate student I liked having a record of what I had run, and an easy way tore-run any single job without needing to run my submission script again. Before we make a job file, let me show you what it looks like:
Importantly, notice the last line! It’s just a single line that calls our script to run our job. In fact, look at the entire file, and the interpreter at the top - #!/bin/bash
- it’s just a bash script! The only thing that makes it a little different is all of the #SBATCH
commands. What’s that about? This is actually thesyntax that SLURM understands as a configuration argument for your job. It just corresponds with the way that you submit the job to slurm using the sbatch
command.In fact, you are free to write whatever lines that you need after the SBATCH
lines.You can expect that the node running the job will have all the same informationthat you have on a login node. This means it will source your bash profile, and know the locations of your $HOME and $SCRATCH. It also means that you can run the samecommands like module load
if you need special software for the job.
What do all the different variables mean?
Some of the above are obvious, like --mem
corresponds to memory in GB, --time
inthe format above means 2 days, and the output and error correspond to file paths to write output and error to. For full descriptions of all the options, the best source is the man pages (linux manual) which you can read via:
If you just had the one job file above, let’s say it were called LizardA.job
, you would submit it like this to slurm:
If it falls within the node limits and accounting, you will see that the job is submit. If you need a helper tool to generatejust one template, check out the Job maker that I put togethera few years ago.
Strategy 1: Submit Directly to sbatch
What if you had the script RScript, and you didn’t want to create a job file? You can do the exact same submission using sbatch directly:
and then of course you would need to reproduce that command to run it again. This is why my preference is for writing a job file. But then it follows that if we have hundreds of jobs to submit, we need hundreds of files. How do we do that?
Write a Loop Submission Script
Here I will show you very basic example in each of R, Python, and bash to loopthrough a set up input variables (the subject identifier to derive an input path)to generate job files, and then submit them. We can assume the following:
- the number of jobs to submit is within our accounting limits, so we will submit them all at once (meaning they get added to the queue).
- at the start of the script, you check for existence of directories. Usually you will need to create a top level or subject level directory somewhere in the loop, given that it doesn’t exist.
- you have permission to write to where you need to write. This not only means that you have write permission, but if you are writing to a shared space, you make sure that others will too.
Bash Submission
Bash scripting is the most general solution, and we will start with it. Here is a basictemplate to generate the SBATCH script above. Let’s call this script run_jobs.sh
Notice that we are echoing the job into the $job_file
. We are also launching thenewly created job with the last line sbatch $job_file
.
Python Submission
With Python, You basically need to do the above, but print to a file using Python.There are multiple ways to do this, here is one example!
The last line submits a job by sending the command to the console. Note that if youwant to do this function in actual software, you would want to use subprocess.
R Submission
This example shows using a relative path to the job file, alongwith printing $SCRATCH as a variable in the file instead of the actual path.
For the below, we are going to use “sink”, which is just a lazy man’s way to write to file, and then the output of cat goes into the file. You can also use cat and specify the file=”” argument.
Again,I recommend NOT doing this programatically first when testing, but to do manual runs first.
Good Practices
Finally, here are a few good job submission practices.
- Always use full paths. For the scripts, it might be reasonable to use relative paths, and this is because they are run in context of their own location. However, in the case of data and other files, you should always use full paths.
- Don’t run large computation on the login nodes! It negatively impacts all cluster users. Grab a development node with
sdev
. - Think about how much memory you actually need. You want to set a bit of an upper bound so a spike doesn’t kill the job, but you also don’t want to waste resources when you (or someone else) could be running more jobs.
- You should generally not run anything en-masse before it is tested. This means that after you write your loop script, you might step through it manually (don’t be ashamed to open up an interactive R or Python console and copy paste away!), submit the job, ensure that it runs successfully, and inspect the output. I can almost guarantee you will have a bug or find a detail that you want to change. It’s much easier to do this a few times until you are satisfied and then launch en-masse over launching en-masse and realizing they are all going to error.
And as a reminder, here are some useful SLURM commands for checking your job.
Do you have questions or want to see another tutorial? Please reach out!
Cluster Computing - Series
This series guides you through getting started with HPC cluster computing.
Python Job Runner Tutorial
Please enable JavaScript to view the comments powered by Disqus.×Share
Share this page with your community.
Comments are closed.